Understanding AI World Models
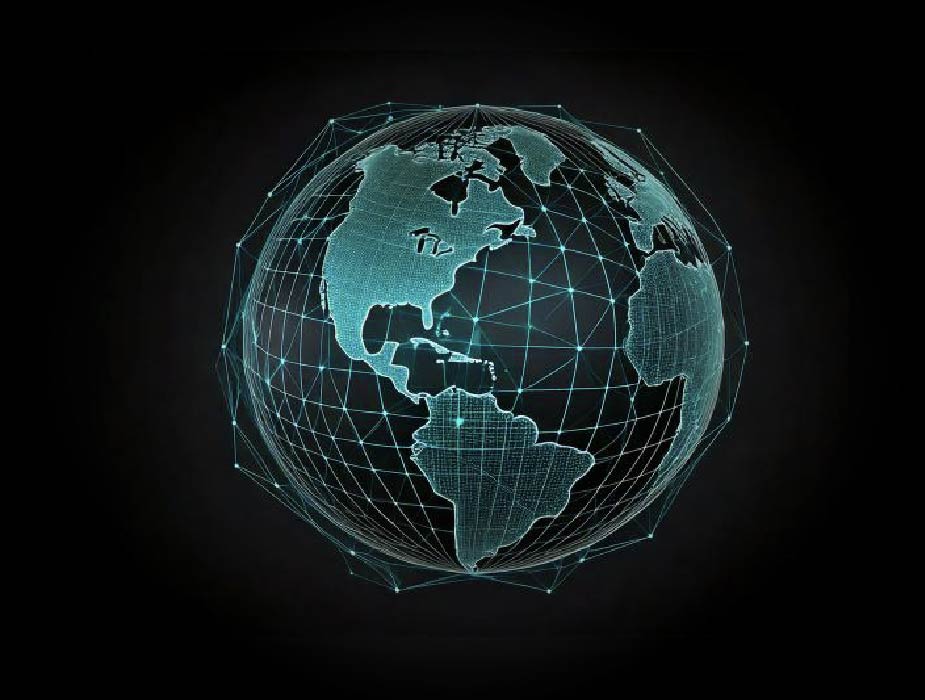
The rapid evolution of artificial intelligence is unveiling a transformative concept called AI world models, which holds promise for achieving a new level of machine understanding and responsiveness that resembles human cognition.
AI world models enable systems to build internal frameworks that mirror how humans process and interpret the world, allowing for nuanced, context-driven insights and interactions. Unlike traditional task-specific models, AI world models create a “mental map” that helps the AI make predictions, interact naturally, and reason through complex scenarios.
This approach is gaining momentum, with major projects like Fei-Fei Li’s World Labs securing $230 million to advance large-scale AI world models and DeepMind’s strategic recruitment of leading AI experts to propel this research.
These models address key limitations of AI, enabling interactions that feel lifelike and intuitive.
For instance, the models are inspired by human cognitive processes, allowing AI to predict outcomes or actions by developing a layered understanding of real-world dynamics—similar to a seasoned athlete predicting the trajectory of a fastball through subconscious reasoning.
Data Fusion and AI world models
The training process for AI world models requires synthesizing data across multiple types—images, audio, video, and text—to form a robust, multidimensional representation of the world. This integration process, called “data fusion,” is what empowers AI systems to interpret scenarios with a rich depth of understanding, leading to more lifelike interactions and responses.
For example, AI world models could significantly reduce the “uncanny valley” effect often seen in video generation, where unnatural details create a jarring experience. Instead, world models simulate interactions that respond and adapt to real-world physics, allowing AI to generate virtual content that feels both accurate and natural.
Beyond video generation, AI world models have significant potential in robotics, digital planning, and other real-world applications. Experts like Meta’s Yann LeCun envision using these models to solve complex, dynamic tasks, such as organizing a room.
Rather than following rigidly programmed instructions, an AI with a world model would infer and act based on context, enabling more natural, adaptive behavior. This capability could transform robotic interactions within human-centered environments, enhancing their utility in spaces like healthcare, logistics, and daily household tasks.
Yet, while AI world models hold tremendous potential, they also face substantial challenges. Training and running these models require vast computational resources, and there’s a risk of generating “hallucinations” or biases due to unbalanced training datasets.
Ensuring the ethical and accurate performance of AI world models is critical, requiring careful oversight and rigorous validation methods.
What Are AI World Models?
AI world models, often referred to as world simulators, are emerging as a groundbreaking concept in artificial intelligence. These models are gaining traction among experts, with significant investments like the $230 million raised by Fei-Fei Li’s World Labs aimed at developing "large world models." Similarly, DeepMind has recruited key figures from the AI community, including a creator of OpenAI's video generator, Sora, to advance research in this area. But what exactly are these world models, and why are they pivotal for the future of AI?
The Inspiration Behind World Models
At their core, world models draw inspiration from humans' mental frameworks to comprehend their surroundings. Our brains naturally create abstract representations based on sensory input, which evolve into concrete understandings of the environment. This cognitive process produces internal models that influence our perceptions and actions. For instance, a study by researchers David Ha and Jürgen Schmidhuber illustrates how a baseball player can predict the trajectory of a fast-moving ball in mere milliseconds—faster than visual signals can reach the brain. This instinctual ability allows players to react without conscious deliberation, relying instead on their internal model of the game.
The Importance of Subconscious Reasoning
The significance of these subconscious reasoning capabilities is underscored by their potential role in achieving human-level intelligence in AI systems. While world models are not new, their relevance has recently surged due to promising applications in generative video technology. Current AI-generated videos often fall into the "uncanny valley," where oddities disrupt realism—like limbs twisting unnaturally or objects behaving contrary to physical laws. A well-developed world model could enhance video generation by providing a deeper understanding of physical interactions, thereby producing more coherent and believable content.
Training World Models
Training these models involves analyzing diverse data types—photos, audio, video, and text—to create comprehensive internal representations that facilitate reasoning about actions and their consequences. Experts like Alex Mashrabov emphasize that viewers expect virtual worlds to behave consistently with reality; deviations can break immersion. Hence, world models can automate object behavior predictions, alleviating creators from tedious manual definitions.
Beyond Video Generation: Broader Applications
Beyond video generation, researchers envision broader applications for world models in sophisticated forecasting and planning across digital and physical domains. For instance, Yann LeCun from Meta envisions a future where an AI equipped with a world model can devise strategies to achieve specific goals—like cleaning a room—by understanding the underlying principles of cause and effect rather than just recognizing patterns.
Challenges Ahead
Despite their potential, significant technical challenges remain. Training world models demands immense computational resources compared to traditional generative models. Moreover, these systems face issues such as hallucination—producing inaccurate outputs—and bias from skewed training data. To overcome these hurdles, researchers must ensure that training datasets encompass diverse scenarios while allowing for nuanced understanding.
The Future of World Models
If successful, world models could bridge the gap between AI and real-world applications more robustly than current technologies allow. They hold promise not only for enhanced virtual environments but also for advancements in robotics and intelligent decision-making systems.