Join our newsletter
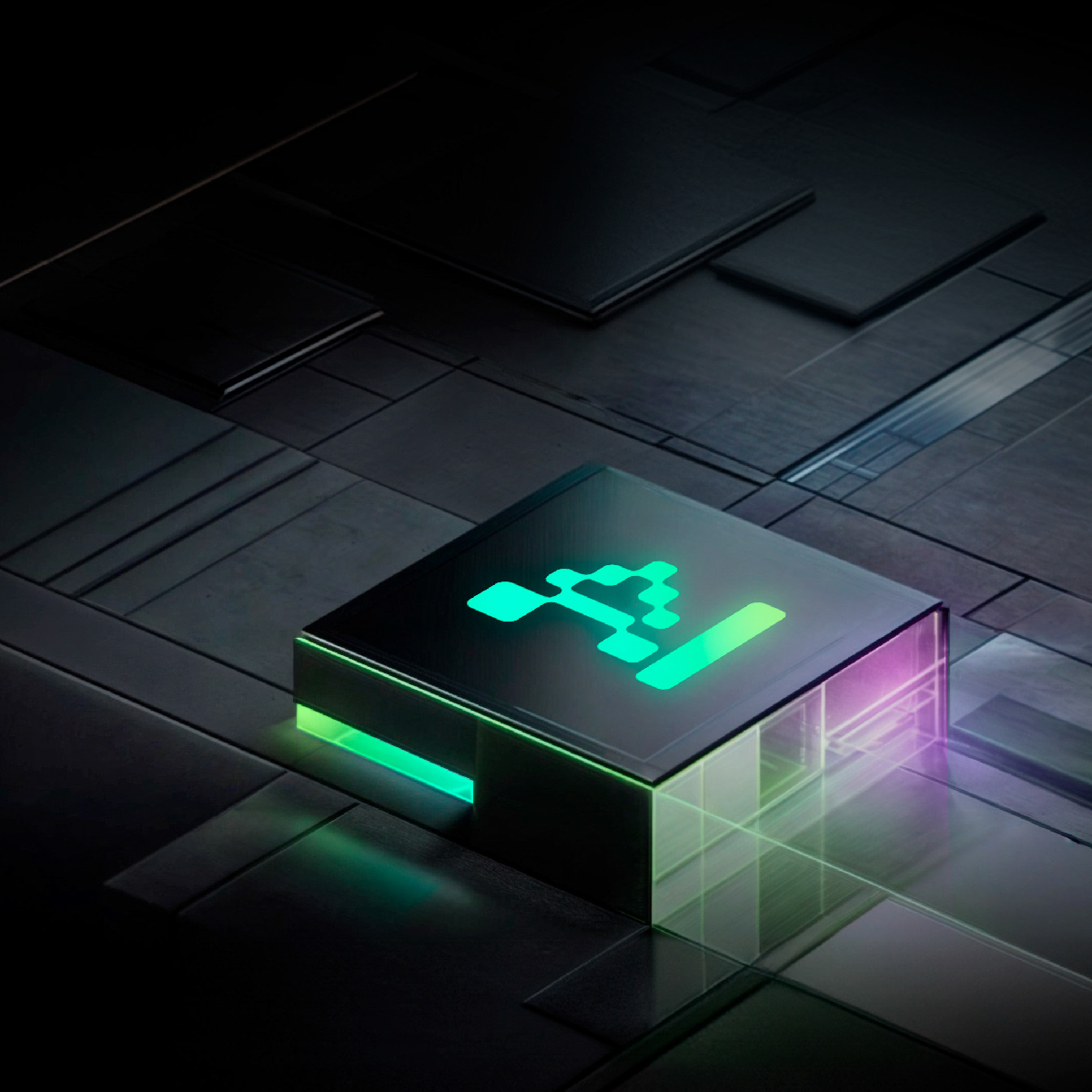
Although AI may sound like the newest thing to hit the street, its origins can be traced back to the 1950s when Alan Turing first posed the question, "Can machines think?" Decades of growth have made AI a vast domain where there can be countless tools and systems. But each individual may wonder, after all, which one is most suitable to the business?
AI has so many different paths, however, none of them are panaceas. The subsets including generative AI, deep learning, natural language processing (NLP), and machine learning (ML) perform distinct functions. The understanding of these technologies will allow a company to trace user problems, optimize the budget, and add artificial intelligence to their workflow. This guide will assist you in selecting a model that fits your needs.
The starting point for taming the powers of AI is often joining hands with some experts trained to negotiate the complexity of the ecosystem. Here, we break down some major AI subsets and their business applications.
Generative AI allows machines to create truly human-like works of content, usually including but not limited to text, images, or complete business proposals. Examples like ChatGPT can help marketers draft copy, develop images, and brainstorm product ideas which can save time and improve efficiency.
Use Cases
Creation of eco-friendly or budget-oriented captions for Instagram posts.
The automated generation of detailed direction for legal documents, via the Thomson Reuters’ CoCounsel program.
Challenges
Hallucinations: The AI can issue diverse and/or fictitious information, so human oversight is a must.
Ethics: Use and abuse of such technologies, like creating deepfakes, present risks to reputation. Clear standards and tools for misuse detection must be constructed.
NLP is a type of AI that allows it to read and respond to language as humans do, making it appropriate for chatbots, sorters of emails, and translators. Businesses deploy it to ease customer interactions, among other things.
Use Cases
Sorting emails into categories -say “client inquiries” or “vendor updates.”
Tracking customer feedback for trends to improve services.
Some of the Common NLP Models Include:
N-gram Models: Predict the next word in a given sequence when typing. For instance, finish “return pol...” with “return policy”.
Neural Network Models: Used for any kind of pattern detection and sentiment analysis within texts.
Transformer Models (LLMs): More advanced tools like GPT-3 can look into the reporting with summarization or content personalization.
Challenges
Language Ambiguity: Often semantic, as in the word "trunk" which has many meanings. Train such models on industry-related data.
Multilingual Barriers: Generally fail with less common languages, which need dedicated datasets or pre-trained models.
Deep learning is analogous to the way a human brain processes data, which makes it very suitable to identify patterns in complex data such as images or videos.
Use Cases
Convolutional Neural Networks (CNNs): Detect and improve quality by identifying defects in images.
Recurrent Neural Networks (RNNs): Observe patterns over periods, like market data for price-making decisions.
Reinforcement Learning: Predict when manufacturing equipment will require maintenance to reduce downtime.
Challenges
Data Quality — Garbage In Garbage Out: Models trained on poor data yield poor predictions, such as missing seasonal trends in retail planning.
Discrimination: Matching text data to another pair of text data can lead to biased data that has a discriminatory impact.
Blackbox: Deep learning-type models act like black boxes so you need tools like SHAP to get trust by explaining them.
Machine learning is about finding the patterns in the data and making decisions. ML employs simple algorithms like linear regression (or even decision trees) as opposed to deep learning.
Use Cases
Analysis of user behavior to predict customer churn on subscription services
Demand forecasting by analyzing items for retail business optimization
Common ML Models
Supervised Learning: Predict future events (e.g., sales trends) based on historical, labeled data.
Unsupervised Learning: It segments customers based on their behavior, allowing for targeted marketing campaigns.
Reinforcement Learning: Uses trial and error to find optimal actions, e.g., by minimizing delivery times.
Challenges
Scale: Innovative model performance over time is automated monitoring and maintenance as models become stale as businesses grow.
Data ethics: In the context of hiring or credit scoring, it's important to find the right balance between fairness and profitability.
All the successful AI models built today have one thing in common: Data. The dataset needs to be clean, accurate, and diverse to get reliable results and to avoid bias. To test the performance of your model on the unseen data and how well it has learned from the training data to identify the areas of improvement, it is a good practice to split your data into training and testing data (i.e., 80/20).
Utilizing external data sources can further improve predictions. For instance, a retailer studying sales trends might include economic data or weather forecasts for a full picture.
Each AI model shines in different areas, and they are:
Generative AI is particularly well-suited to automating creative functions such as content generation.
NLP is effective in comprehending human language and improving customer interaction.
Deep Learning deals with such complex problems as pattern recognition in photos or videos.
Machine Learning is great for data-driven insights, like demand forecasting.
The key to success is a combination of high-quality data with a top-tier development team. This will allow your business to unlock AI's potential for smarter decision-making and sustained growth.
For more information on AI, contact our sales team at sales@kenility.com and learn how AI can transform your business.