Join our newsletter
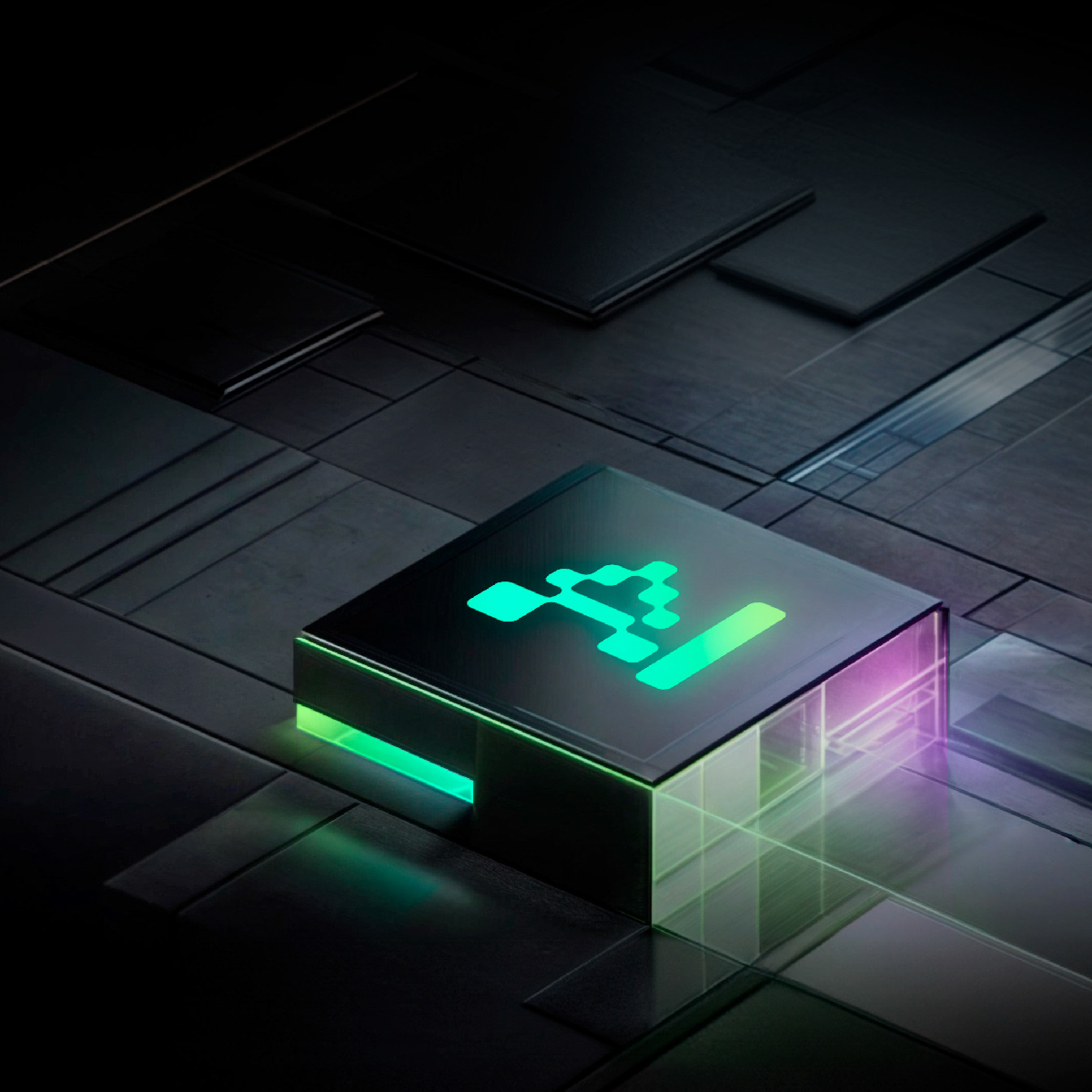
"Systems engineering is entering a new era. AI-driven automation is no longer just improving efficiency—it’s reshaping the way complex systems are designed, tested, and maintained."
Traditional systems engineering relies on meticulous planning, iterative testing, and extensive manual processes. However, as systems grow more complex, AI-driven automation is becoming essential for optimizing workflows, minimizing errors, and accelerating development cycles.
By integrating machine learning, real-time data analytics, and intelligent automation, AI is enhancing decision-making, reducing engineering bottlenecks, and enabling self-optimizing architectures that adapt in real time.
Model-Based Systems Engineering (MBSE) relies on digital models to define, analyze, and optimize system behavior. AI enhances MBSE by automating design validation, predicting system performance, and suggesting optimizations based on real-time simulations.
AI-driven automation eliminates time-consuming manual testing by simulating thousands of test scenarios in seconds, identifying edge cases, and ensuring system resilience under dynamic conditions.
AI enables self-optimizing systems that automatically adjust performance parameters based on real-time data. This is particularly valuable in industries like manufacturing, logistics, and smart infrastructure.
Machine learning models analyze sensor data to predict system failures before they occur, allowing for proactive maintenance and reducing costly downtime.
While AI is revolutionizing systems engineering, its adoption comes with challenges:
AI is not replacing engineers—it is enhancing their ability to design, test, and optimize complex systems with greater speed, precision, and adaptability. Organizations that adopt AI-powered systems engineering will gain a competitive edge, reduce costs, and drive innovation at an unprecedented scale.
At Kenility, we help businesses integrate AI into systems engineering, optimizing workflows, accelerating development, and ensuring high-performance, resilient, and adaptive system designs.
🚀 Is your organization ready for AI-driven systems engineering? Let’s talk. Contact us at sales@kenility.com