Join our newsletter
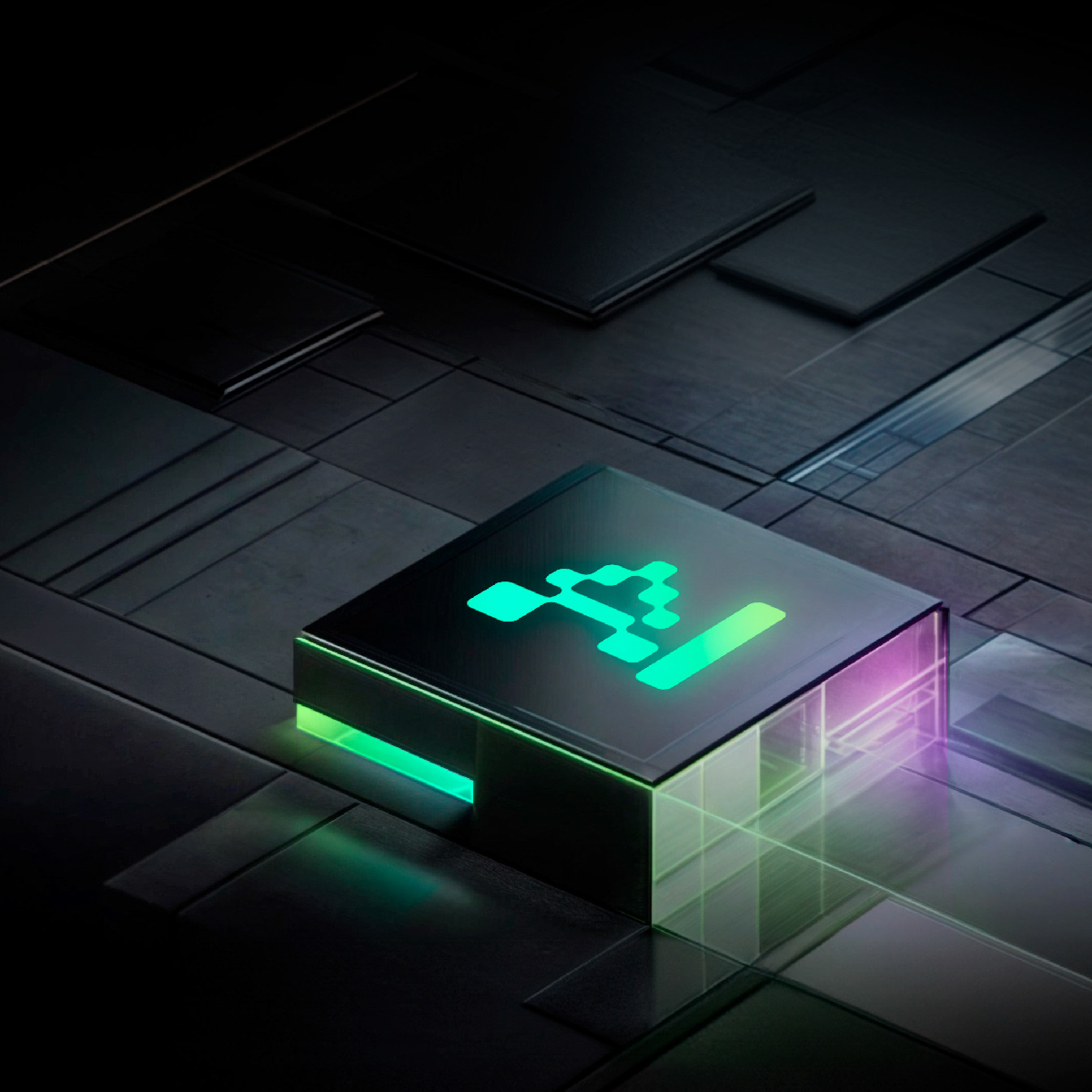
In today's competitive business landscape, predictive analytics has evolved into a crucial tool for driving strategic decisions and achieving sustainable growth. For CTOs and CEOs, leveraging predictive analytics can translate into smarter resource allocation, improved customer engagement, and ultimately, a stronger bottom line. With advancements in data science, machine learning, and computational power, predictive analytics in 2024 offers more accurate, scalable, and actionable insights than ever before. This article delves into how top-level executives can harness predictive analytics to power business growth, supported by statistics and best practices.
Predictive analytics uses historical data, machine learning algorithms, and statistical models to forecast future outcomes, uncover trends, and identify opportunities and risks. Companies utilizing predictive analytics see significant improvements in efficiency, customer retention, and overall business growth.
According to recent research from Allied Market Research, the global predictive analytics market is projected to reach $35.45 billion by 2027, growing at a CAGR of 21.9% from 2021 to 2027. This high adoption rate underscores its role as a core business driver.
For CTOs and CEOs, implementing predictive analytics isn't just about staying competitive; it's about reshaping business operations to be proactive and insights-driven. As an example, McKinsey reports that organizations using predictive analytics can see ROI improvements ranging from 10-30% on marketing expenditures alone.
How It Works: Predictive analytics enables companies to analyze customer behavior, such as purchase history, browsing patterns, and engagement levels, to create highly personalized experiences. Machine learning models can identify patterns in customer data, segmenting audiences with remarkable precision.
Impact on Growth: According to a study by Boston Consulting Group, personalized marketing driven by predictive analytics can increase revenue by 10-20%. For instance, predictive models can enhance customer lifetime value (CLV) by forecasting individual purchasing behaviors, and enabling targeted offers that boost conversion and retention rates.
How It Works: Sales forecasting using predictive analytics enables companies to anticipate demand and optimize supply chains, minimizing wastage and enhancing efficiency. By analyzing historical sales data alongside economic and seasonal factors, predictive models can provide insights into future demand trends.
Impact on Growth: Research by Deloitte found that companies using advanced forecasting techniques achieve a 15% improvement in inventory turnover and a 10% reduction in out-of-stock situations. Predictive sales forecasting reduces supply chain bottlenecks, minimizes stockouts, and ensures that companies meet customer demand in real time.
How It Works: Predictive models can analyze vast amounts of transaction data to detect anomalous patterns indicative of fraud or security breaches. Machine learning models in risk management provide real-time alerts, helping businesses prevent potential losses and security threats.
Impact on Growth: According to IBM, businesses using predictive analytics for fraud detection experience a reduction in fraudulent activity by up to 50%. For financial services, predictive models in risk management not only reduce fraud but also ensure regulatory compliance, significantly impacting business continuity and trust.
How It Works: Predictive analytics can analyze machine performance, staffing requirements, and production outputs to streamline operations. For example, predictive maintenance models assess equipment data to anticipate failures, thus minimizing unplanned downtimes and reducing maintenance costs.
Impact on Growth: A report by McKinsey shows that companies using predictive maintenance techniques can reduce maintenance costs by 10-40% and downtime by up to 50%. For industries reliant on heavy machinery, such as manufacturing and logistics, predictive analytics improves operational uptime, directly impacting profitability.
Predictive analytics is an indispensable tool for achieving data-driven business growth in 2024. By understanding and forecasting future trends, CTOs and CEOs can make informed decisions that align with organizational goals and enhance competitiveness. With predictive analytics, organizations can anticipate and respond to changes in market dynamics and build a proactive, data-first culture.
Investing in the right tools, skilled talent, and a strategic approach to predictive analytics ensures that businesses can leverage data as a powerful growth catalyst. As predictive technologies continue to evolve, businesses that harness their full potential will gain a critical advantage in today's digital economy.