Join our newsletter
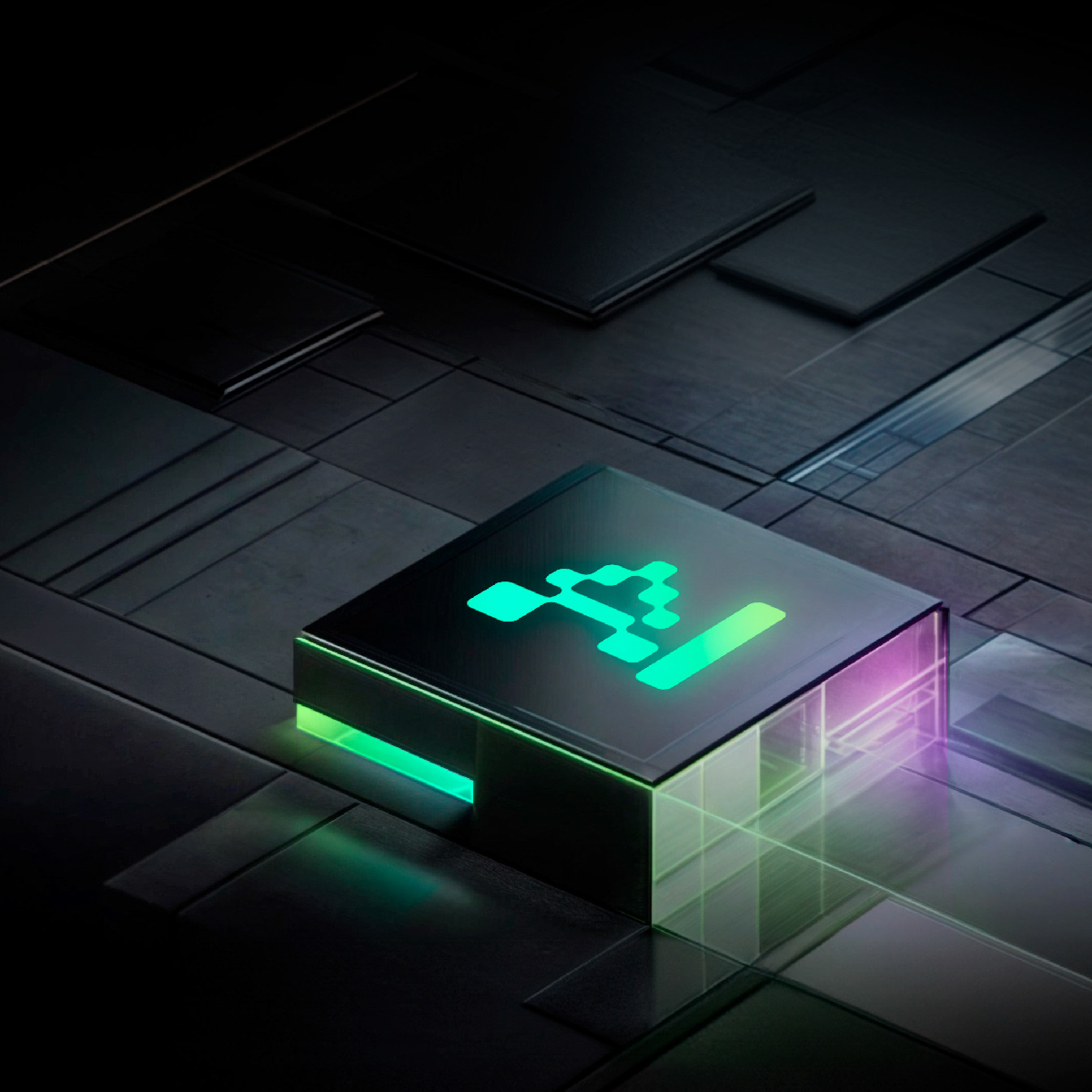
Key Takeaways for Successful Data Preparation
Good Data is Essential: Generative AI (genAI) relies heavily on relevant, accurate, and accessible data to deliver successful outcomes.
Ongoing Data Management: Data preparation is not a one-time task but a continuous process requiring regular updates and quality checks.
Comprehensive Data Inventory: Create a complete catalog of all data sources to manage and organize information effectively.
Quality and Governance: Establish data quality standards and governance policies to ensure accuracy, security, and compliance.
Avoid Common Pitfalls: Address incomplete datasets and unstructured data to enhance genAI effectiveness and insights.
Generative AI (genAI) applications thrive on relevant, accurate, and highly accessible data, yet preparing that data can be complex and requires ongoing effort. CIOs face increasing pressure to implement AI initiatives that boost organizational competitiveness, efficiency, and productivity. However, many organizations find data management as challenging as it is crucial.
At Gartner’s London Data and Analytics Summit earlier this year, Senior Principal Analyst Wilco Van Ginkel forecasted that at least 30% of genAI projects would be abandoned after the proof of concept phase through 2025, citing ∫ as a major factor. Senior Director Analyst Roxane Edijlala also highlighted that "having data ready for AI drives greater business outcomes by 20%."
Many businesses struggle with preparing their data for genAI, concerned that the process will be overwhelming, especially for those lacking in-house data or AI expertise. Organizations need to recognize that the efficacy of AI is directly tied to the quality of the data it uses.
Good data must be well-prepared, manageable, and accessible across various environments where genAI tools are implemented.
To leverage genAI effectively, organizations need comprehensive access to all their data. This requires managing vast amounts of information in both physical and digital formats, often spread across complex enterprise systems and numerous data silos. The first step is to create a complete inventory of all data sources, detailing their locations and formats. This inventory serves as a foundation for organizing and managing the data.
Next, assess and improve data quality by setting standards for accuracy, completeness, and reliability. IT teams can use these standards to identify and rectify issues in existing data and apply them to incoming data for ongoing quality management.
Implementing robust data governance and security measures is crucial to protect against breaches and ensure regulation compliance. This includes setting up effective governance tools and clear data retention schedules. It’s also important to ensure data is sourced legally and ethically, respecting privacy and intellectual property rights.
Common pitfalls in data management include not maintaining a comprehensive dataset and failing to address both structured and unstructured data. Often, only about 20% of required data is readily visible, while 20% may be redundant, obsolete, or trivial. The remaining 60% is typically unstructured, such as paper documents or siloed systems, and includes valuable communication data like audio, video, and chat.
Failing to make this unstructured data visible and accessible limits the potential of genAI, hindering the ability to gain meaningful insights and train algorithms effectively. Instead of trying to handle everything at once, focus on a specific use case with fewer data sources and formats to streamline the process.
By investing time in setting up effective data management processes, organizations can ensure their data supports successful genAI initiatives, paving the way for enhanced productivity and innovation.